Rating AI System
ABSTRACT
Advancements in Artificial Intelligence (AI) technologies have been applied to almost every aspect of Customer Experience Automation. Improving customer satisfaction is one of the end goals of these applications. AI solutions also provide capabilities to measure and analyse customer satisfaction, with advantages such as capturing interactions in real time, big data analysis on behaviour and sentiments, multi-channel survey feedback and many other use cases. Few AI solutions however, address the historical issue of improving response rates to customer surveys, without which the effectiveness of understanding customer satisfaction is diluted.
Human Pixel’s DiNA Rating AI, takes an innovative approach to helping businesses measure customer satisfaction. It makes use of machine learning AI algorithms to predict ratings of interactions, where the customer has not responded. By taking a predictive approach instead of reactive analysis and by expanding the scope of customer feedback to cover “the silent majority”, DiNA Rating AI provides valuable benefits for businesses to understand the critical KPIs of customer satisfaction and customer (service) effort.
INTRODUCTION
Traditionally surveys have been the main feedback tool used to capture and understand the ratings of customer interactions and the customer service industry has struggled to improve response rates for customer satisfaction surveys which average between 5-40% depending on several factors such as survey design, channel, process, intent etc. This leaves out feedback from a large segment of:
a) dissatisfied customers who had a negative service interaction and have stopped engagement, or;
b) happy, loyal/repeat customers who may have no incentive to contribute their feedback.
Advancements in AI technology have made it possible to perform and even predict ratings of customer interactions. However, many AI solutions apply complex solutions such as real-time sentiment monitoring, big data analysis etc. which still leave out the “silent majority”.
Human Pixel’s Rating AI addresses this problem. Human Pixel’s Rating AI is an Artificial Intelligence solution that automates performance ratings from customers for interactions with customer service agents, both human and virtual. Named DiNA, the AI is a multi-output classifier that has been “trained” on previously rated customer interactions. By using a trained machine learning algorithm, businesses can rate interactions for which their feedback was not received or were not rated by customers directly. The predicted results allow for real time monitoring of agents that are underperforming as well as the reasons for unsatisfied customers.
The ratings AI can be used to monitor the performance of human agents (or DiNA), in real time. Chats are rated by customer on combined scoring of four different parameters Relevance, Empathy, Resolution and Overall Experience. The end outcome is an objective performance measure over and above “sentiment”.
WHAT IS RATING AI
Human Pixel Rating AI is not automation of surveys; it is automation of rating for unrated customer interactions. For example, customers for a business may complete survey results on a small fraction of interactions (10%). The business will not have visibility into the remaining 90% of contacts. This generates blind spots which might be detected weeks or months after occurring. Further, human analysis and interpretation of the available feedback is time consuming, subjective and prone to bias. Neither it is possible to efficiently review and analyse every interaction in real time.
The core proposition with DiNA is to leverage AI technology to analyse large amounts of customer data which would be impossible for humans to process in real time. Further, the AI model has the capability to rate interactions that are not rated by customers so that the company has complete operational visibility into the customer experience.
DiNA can be configured to rate interactions based on company specific survey questions. The rating results are driven by on the survey questions. Human Pixel has trained the rating AI on the following survey set which captures the key performance indicators of customer experience: overall result, empathy, relevance, and resolution. Human Pixel can train the algorithm based on the survey questions specific to client requirements.
HOW RATING AI WORKS
The figure above provides an overview of how Human Pixel Rating AI (DiNA) works. Customer survey ratings are fed into DiNA, our rating AI, which is a multi-output classifier that’s been “trained” on previously rated customer interactions such as overall result, empathy, relevance, resolution amongst others. DiNA uses the information and learnings on the new untrained chat to provide a new rating.
DiNA also has the ability to “multi-task learning” and is independent of any human sentiments that can potentially influence traditional methods.
Analytics Insights
DiNA Rating AI also offer analytics insights into the rating process through real time performance monitoring reports and dashboards. The data can be streamed to customers for data visualizations within their own call centre monitoring software or business dashboards. Data visualisations can be implemented on any standard platforms such as Domo, Tableau, Power BI, Oracle etc.
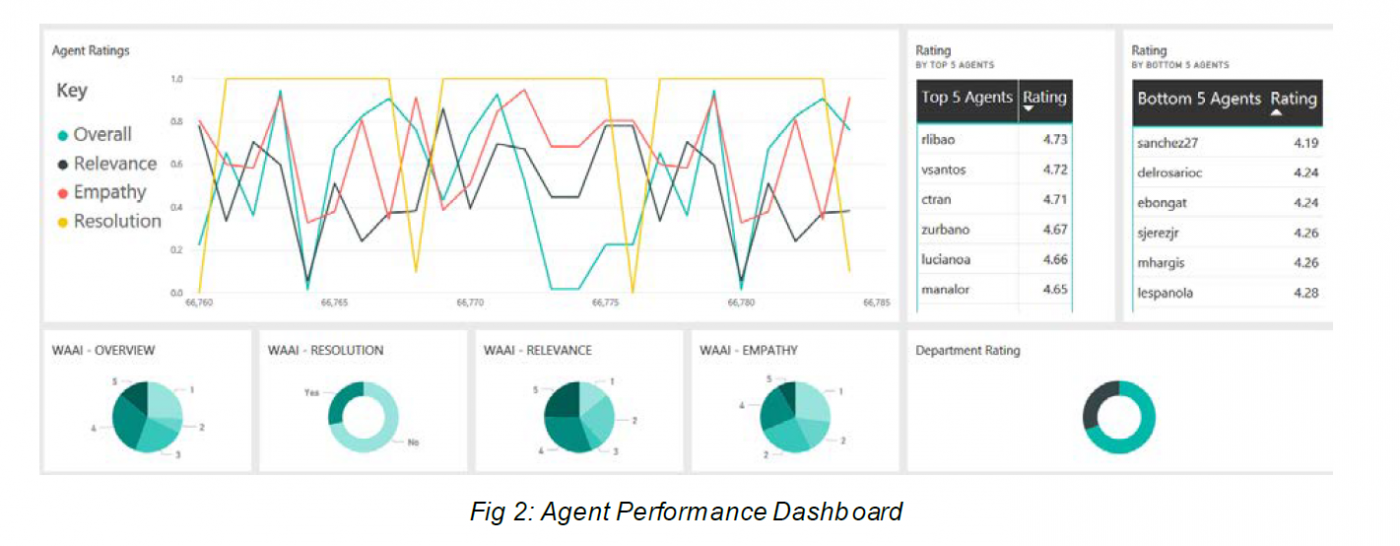
RATING AI MODEL OPERATION: TESTED ON RATED CHARTS
Human Pixel AI built a deep learning model with convolution, max pool, fully connected layers. The data was split into 80% for training, and 20% for test. To avoid overfitting, instead of oversampling the data which makes the model learning slower, we adjusted the loss function to reduce the impact of majority class in each learning epoch. The model will learn to adjust the parameters based on this loss to increase the accuracy.
The data consisted of 14,113 rated chat content. The longest chat had 1,849 words. Each chat has 4 ratings Q1, Q2, Q3, Q4. The following charts show the value distribution in these ratings. The data available for the test was somewhat skewed towards higher, affirmative ratings. Majority of chats had been rated as 4 or 5 for Q1, Q2 and Q3, as “Yes” for Q4.
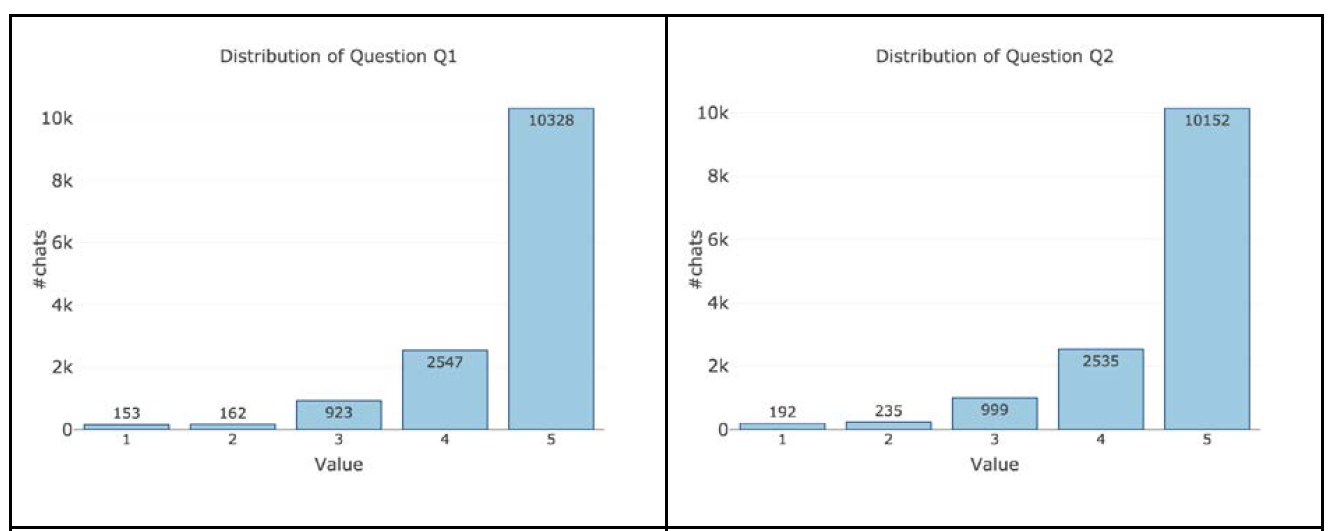
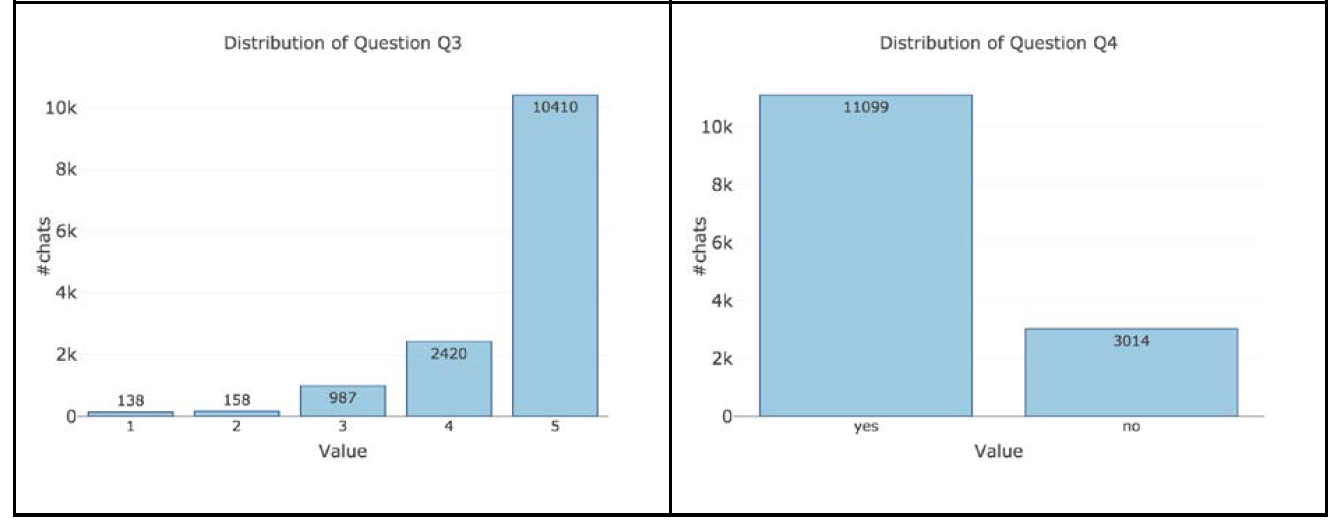
However, if we have a less skewed distribution of data like the real world, the model will be better in prediction for real chat content. The performance on Q1, Q2, Q3 and Q4 questions on the test set were as under.
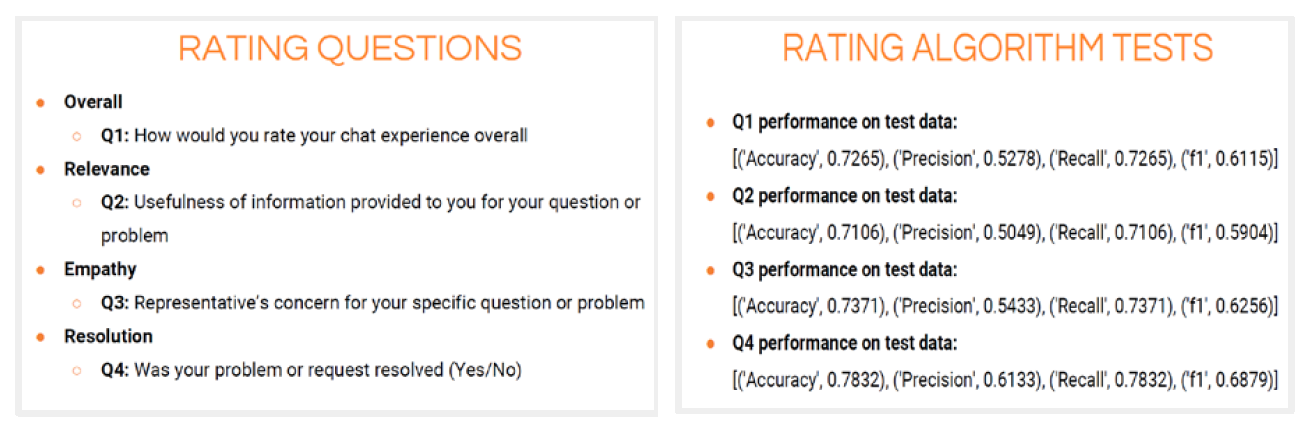
- Expanding Operational Visibility into Customer Satisfaction
Several metrics for measuring customer experience and engagement exist such as CSAT (Customer Satisfaction), NPS (Net Promoter Score), Customer Effort Score (CES) etc. Using Rating AI, these KPI’S can be used to cover both rated and unrated interactions.
The Rating AI provides insight into unrated customer interactions. By training the AI on a history of current and past interactions, the model can predict ratings for non-responded surveys after a customer interaction. The business can get insights on customer satisfaction over a wider scope, potentially covering 100% interactions. - Monitor agent performance
DiNA Rating AI can flag agents for non-performance and training. Under-performing agents can be coached on deficiencies via the ratings data. Conversely, the system also enables recognition of top performers and the ability to reward high performing agents. - Follow-up with dissatisfied clients
Ratings prediction can signal clients who were dissatisfied with the service interaction. The business can promptly follow-up with the clients to resolve their issue. - Referrals from satisfied customers
Pro-active follow-up on interactions even where unrated, conveys to the client that their needs are being looked after. Thus, customer sentiment can be changed from neutral or negative to positive. These clients turn into satisfied customers and the business receive referrals from them. The business can also improve customer lifetime value by gaining customer loyalty and upselling/cross-selling other products and services. - Improve Training Efficiency
The Rating AI can recommend chats to be used in training agents, human as well as DiNA. It separates quality chats from training sets or from self-learning, improving training data and increasing training efficiency.
DIFFERENTIATION OVER “SENTIMENT ANALYSIS” TOOLS
Sentiment analysis tools are designed to detect customer sentiment in an interaction measuring attributes such as tone, negative keywords and so on. These tools have shortcomings when compared with rating AI. They are non-granular and non-specific and can be applied to over a wide context, making objective analysis difficult, unless examined individually. They are not trained on customer feedback or history of customer’s interaction with the business. Sentiment tools may also not correlate with survey responses. Finally, sentiment cannot be used as an indicator of agent performance, a key business objective in AI work assignment and overall customer service KPI.
KEY CONSIDERATIONS FOR RATING AI
- The distribution of questions is key and the data for training should ideally follow a normal distribution curve. If many questions skewed and always elicited as 4 or 5, this can have an impact on the rating.
- Ensuring the data is clean and relevant is also important. Data which has lots of “noise” can impact the ratings.
- Rating results depend on the survey questions and hence a properly designed survey result is essential to increasing accuracy.
- Accuracy is higher if the number of survey questions is lower. Optimally 4 to 5 questions are recommended for a better result and higher customer response.
HOW HUMAN PIXEL CAN HELP
DiNA Rating AI can be quickly deployed as an on-premise solution supporting soft integration with existing rating data. Our AI experts will help in understanding the current model and provide a roadmap on implementation.
In general, implementing a new model requires up to 10,000 records for the training set. We need access to the rating data and the chat/call/ticket content data. We also need endpoints for pulling live data and for pushing results data